A public mid-density genotyping platform for alfalfa (Medicago sativa L.)
Abstract
Small public breeding programmes have many barriers to adopting technology, particularly creating and using genetic marker panels for genomic-based decisions in selection. Here we report the creation of a DArTag panel of 3,000 loci distributed across the alfalfa (Medicago sativa L.) genome for use in molecular breeding and genomic insight. The creation of this marker panel brings cost-effective and rapid genotyping capabilities to alfalfa breeding programmes. The open access provided by this platform will allow genetic data sets generated on the marker panel to be compared and joined across projects, institutions and countries. This genotyping resource has the power to make routine genotyping a reality for any breeder of alfalfa.
Keywords
Alfalfa, amplicon-sequencing, plant breeding, DArTag genotyping, microhaplotype
Introduction
Molecular breeding techniques have been used for nearly four decades to enhance and speed breeding efforts for major staple food crops like tomato, maize and barley (Feurerstein, Brown, & Burdon, 1990; Hasan, Choudhary, Naaz, Sharma, & Laskar, 2021; Helentjaris, King, Slocum, Siedenstrang, & Wegman, 1985; Tanksley, 1983). Over time, these techniques have been augmented with high-quality phenotypic data to perform genome-wide association studies (GWAS) and genomic selection and prediction, further fueling breeding for quantitative or complex traits (Eathington, Crosbie, Edwards, Reiter, & Bull, 2007; Heffner, Sorrells, & Jannink, 2009; Lorenzana & Bernardo, 2009). While these achievements are significant, many crop species grown for human consumption and livestock feed are still unable to apply these techniques in breeding efforts. Many breeders would like to adopt molecular breeding tools and techniques, but sometimes doing so is hampered by large barriers-to-entry challenges. The range of barriers and how surmountable they are, varies from species to species and is impacted by species-specific challenges in logistics, technical know-how, biology and the growing environment.
Alfalfa (Medicago sativa L.) is the most widely grown perennial forage crop worldwide (Undersander, 2021). In the United States, it was the fourth most cultivated crop in 2021 with an estimated direct value of US$11.6 billion (Putnam & Meccage, 2022) and ranked first among forage crops planting area with a total of 14.9 million acres in 2022 (https://www.nass.usda.gov/). Alfalfa is a key nutritional component for dairy and beef production because it contains a high amount of crude protein, provides dietary fibre needed to maintain rumen health, and is an excellent source of vitamins and minerals. In addition, it is unparalleled as a component of sustainable agricultural systems because of its ability to fix nitrogen, protect water quality, interrupt pest and pathogen cycles in annual crops, and improve soil carbon storage (Fernandez, Sheaffer, Tautges, Putnam, & Hunter, 2019). Alfalfa is adapted to different growth environments and depending on location and management, is highly persistent.
As a highly heterozygous outcrossing autotetraploid species, alfalfa features a predominant pattern of random chromosome pairing during meiosis. The four sets of chromosomes add a layer of complexity to genotyping endeavours. Traditional SNP marker systems, primarily designed for diploid species, often fall short when applied to alfalfa due to their inability to identify allelic dosages accurately. Thus, the intricacies of alfalfa's genetic structure call for more sophisticated SNP genotyping systems capable of addressing the unique challenges posed by its autotetraploid nature. The investment cost and reliance upon skilled bioinformatics support for each genotyping run, make this a high-risk technology for breeders to adopt.
Currently, most alfalfa cultivars are synthetic populations developed by multiple cycles of phenotypic selection for desired traits. Evaluation for biomass yield, winter survival, grow back, disease resistance and forage nutritional quality among other traits is a multi-year process before cultivar registration and commercial seed production. Breeding programmes have operated with half-sib populations originating from polycrosses, where only the maternal parent is known, and the paternal parent can range from a few individuals up to hundreds. Unfortunately, breeding for yield gains in alfalfa using traditional phenotypic evaluation and recurrent selection methods has hit a plateau partly due to its highly heterozygous and heterogeneous population-level breeding. Community genomic tools like those in the ‘Tools for Polyploids’ project (https://www.polyploids.org/) or developed in other polyploid outcrossing crops have shown promise in accelerating breeding and yield gains (Ferrão, Amadeu, Benevenuto, Oliveira, & Munoz, 2021). The creation of genomic tools that account for the biological and logistic challenges of the crop, has the potential to significantly improve yield gains in alfalfa through breeding.
The first and typically most tractable place to build capacity and tools for molecular breeding is to begin by creating a rapid genotyping pipeline that fits within both the breeding cycle and the selection cycle and can deliver on the breeder’s objectives (Hawkins & Yu, 2018; Mejia-Guerra, Zhao, & Sheehan, 2021). As stated here, a pipeline refers to a complete workflow starting with a genetic marker platform, vendors for service and bioinformatic tools to transform returned raw data into a usable format for breeders. There are several factors to consider when choosing a genetic marker platform: cost per data point, vendor services, turnaround times and what genetic analyses can be done with the resulting data. For alfalfa, we determined that a targeted-amplicon sequenced-based approach would be the most beneficial for breeders. Unlike Genotyping-by-Sequencing (GBS), targeted, amplicon-based genotyping technologies such as DArTag (Diversity Array Technology - DArT), and Capture-Seq (LGC Genomics) have low missing data rates and query the same exact loci in all samples across genotyping projects, allowing new data to be easily appended to existing data (Darrier et al., 2019; Telfer et al., 2019; Wang et al., 2020). The amount of data returned is in the tens of thousands or less, rather than the millions of reads from GBS, simplifying downstream bioinformatics processing (Darrier et al., 2019; Milner et al., 2019). This in turn speeds up the analysis time for marker-assisted selection (MAS), introgression tracking, linkage mapping and genomic prediction (Darrier et al., 2019).
Here, we report the creation of a DArTag panel of 3,000 loci distributed across the alfalfa genome for use in molecular breeding and genomic prediction. DArTag is a hybridization/amplicon-based targeted genotyping platform developed by DArT (Blyton et al. (2023); https://www.diversityarrays.com/services/targeted-genotying/). Oligos are custom designed to target known genetic variants (SNPs and InDels less than 50bp) with its flanking genomic regions, and sequencing products of 54bp (legacy technology) or 81bp (current technology) in length are produced.
The DArTag assay consists of four steps based on principles described in (Krishnakumar et al., 2008). Briefly, the pool of 3,000 alfalfa oligos, each targeting one genetic variant, is hybridized to denatured gDNA in step 1, followed by SNP/INDEL copying into DArTag molecules by DNA polymerase in step 2. After ligation into circular molecule also in step 2, and nucleases treatment to remove unwanted molecules in step 3, DArTag products are subsequently amplified in step 4 with simultaneous addition of sample unique barcode used downstream for demultiplexing. The products of DArTag assay, after purification and quantification, are sequenced on NGS platforms (e.g. NovaSeq 6000, Illumina) to a depth of around 350x per marker per sample, then demultiplexed and genetic variants detected using DArT’s proprietary analytical pipeline.
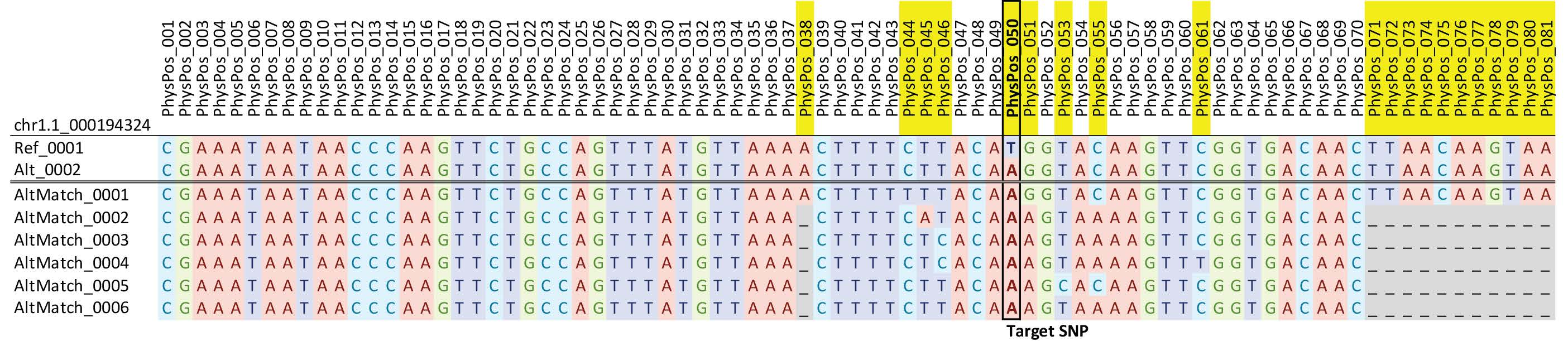
The alfalfa DArTag panel was designed on the legacy technology to produce 54bp reads but works equally well with the current technology (81bp reads) with the caveat that some residual adapter sequences may be included (read-through of the entire fragment into the adapter). After trimming of any residual adapter sequences, the reads can be used to call SNPs, or in the case of complex genomes like alfalfa, used to identify microhaplotypes (Figure 1). Sequencing reads can contain variants beyond the target SNP, which allows for the detection of more than two alleles at each of the 3,000 loci. As the amplicons are very short, variants found within these reads are assumed to be in complete linkage disequilibrium and therefore can be used for phasing genotyping calls for genetic map construction. In addition, accurate allele dosage can be determined for both bi-allelic and multi-allelic haplotypes, allowing genetic effect contributions to be determined for each unique haplotype for traits of interest. As DArT had not tested many polyploid species with DArTag when this study was initiated, we agreed to limit the number of probes to 3,000 loci, though the optimal max may differ by species and genome complexity, and read depth required to sufficiently call genotypes (Andrzej Kilian, DArT, personal communication).
Results
This alfalfa 3K DArTag panel was developed from a diversity panel of 40 individual alfalfa clonal genotypes, focusing on elite breeding and stress-resistant genotypes used in North America. This panel consisted of 17 elite parents with various fall dormancy levels, six samples of diploid-cultivated alfalfa, 13 genotypes with abiotic stress resistance, one genotype with Aphanomyces root rot disease resistance, and three other genotypes (Table 1, column 2). Two biological replicates of the diversity panel were processed, where the sequencing libraries were prepared using either Illumina Nextera WGS library prep at Cornell Institute of Biotechnology or NEBNext Ultra DNA Library Prep Kit with an average insert DNA size of 300bp. The whole-genome sequencing (WGS) was done using Illumina NovaSeq 6000 at Novogene (https://en.novogene.com/). Raw FASTQ sequences were processed by removing residual adapter sequences and low-quality bases using Trimmomatic (LEADING:10 TRAILING:10 SLIDINGWINDOW:4:15 MINLEN:30) (Bolger, Lohse, & Usadel, 2014). Cleaned reads were then aligned to the haploid set (the first set out of the four homologous chromosomes) of XinJiangDaYe reference genome (Chen et al., 2020) using BWA-MEM (Li, 2012; Li, 2013) and structural variants (SNPs and indels) were called using the DNAseq pipeline developed by Sention (https://www.sentieon.com). A total of 28M SNPs present in both replicates were discovered from the whole-genome re-sequencing of the diversity panel, where a high-confidence set of 10K SNPs (Figure 2) were obtained by requiring them: (1) not located within 5bp distance to an indel, (2) QUAL > 30, (3) minimum and maximum read depths of 20 and 1,900, respectively, (4) for each sample, at least one read supporting reference allele and two reads supporting the alternative allele, (5) no missing genotype per SNP position, (6) with a minor allele frequency greater than 0.25, (7) not located in transposable elements and (8) not within 1Kb of chromosome termini. The 10K SNPs were assessed by DArT, and from those that passed QC, a 3K SNP set targeting even genomic distribution was selected to form a 3K DArTag marker panel. Of the 3,000 loci selected for the panel, 85% (2,542) reside in genic regions and only 15% (458) reside in non-genic regions (Supplemental Table 1). Oligo probes were synthesized, and genotyping done at DArT.
The alfalfa 3K DarTag marker panel was validated using a bi-parental F1 population (n = 184), a backcross (BC1) population (n = 94), and a diverse set of elite genotypes (n = 74) and individual plants from other Medicago species (n = 20) (Table 1, column 3). It should be noted that all 40 alfalfa lines used in the SNP discovery were also included in this validation sample set. The material selected for validation was to assess (1) the panel’s ability to construct genetic (linkage) maps with the data output and (2) to define the usable limit to the panel with extant species (non-Medicago sativa) germplasm.
As expected, the missing data (a marker with < 10 reads in a population) is the lowest among the Medicago sativa genotypes. The alfalfa lines used in SNP discovery showed the least missing data (an average of ~9% of the 3K markers with no data) and the rest M. sativa lines of the validation sample set had comparable missing rates (10%) (Supplemental Figure 2). Other Medicago species had an average of 51% markers with missing data, which is approximately five times higher than the M. sativa genotypes.
Sample ID |
SNP discovery |
Validation set |
Contributor |
Note |
---|---|---|---|---|
S&W FD4 |
Y |
Y |
S&W Seed Co. |
Elite parent; fall dormancy 4 |
S&W FD5 |
Y |
Y |
S&W Seed Co. |
Elite parent; fall dormancy 5 |
Legacy FD4 |
Y |
Y |
Legacy Seeds |
Elite parent; fall dormancy 4 |
Legacy FD5 |
Y |
Y |
Legacy Seeds |
Elite parent; fall dormancy 5 |
S&W FD6 |
Y |
Y |
S&W Seed Co. |
Elite parent; fall dormancy 6 |
S&W FD7 |
Y |
Y |
S&W Seed Co. |
Elite parent; fall dormancy 7 |
S&W FD8 |
Y |
Y |
S&W Seed Co. |
Elite parent; fall dormancy 8 |
S&W FD9A |
Y |
Y |
S&W Seed Co. |
Elite parent; fall dormancy 9 |
S&W SFD9B |
Y |
Y |
S&W Seed Co. |
Elite parent; fall dormancy 9 |
CADL-1 |
Y |
Y |
N. Young |
Cultivated alfalfa at diploid level |
CADL-3 |
Y |
Y |
N. Young |
Cultivated alfalfa at diploid level |
CADL-4-5 |
Y |
Y |
N. Young |
Cultivated alfalfa at diploid level |
CADL-5-3 |
Y |
Y |
N. Young |
Cultivated alfalfa at diploid level |
CADL-13 |
Y |
Y |
N. Young |
Cultivated alfalfa at diploid level |
CADL-18 |
Y |
Y |
N. Young |
Cultivated alfalfa at diploid level |
UMN3988-BIP |
Y |
Y |
D. Samac |
Biomass type |
RegenSY27x |
Y |
Y |
D. Samac |
Regenerator; Ref. genome |
I195 |
Y |
Y |
N. Young |
WAPH5; Aphanomyces root rot |
UT14-46 SP |
Y |
Y |
M. Peel |
Tetraploid Medicago falcata |
UT27-62 |
Y |
Y |
M. Peel |
Elite parent; Salt tolerant |
FL99 |
Y |
Y |
E. Rios |
Elite parent; Fall dormancy 9 |
Bulldog 505 |
Y |
Y |
A. Missaoui |
Elite parent; fall dormancy 5 |
GAMS 1403-FSH |
Y |
Y |
A. Missaoui |
Elite parent; fall dormancy 7 |
GAMS 1404-FSH |
Y |
Y |
A. Missaoui |
Elite parent; fall dormancy 8 |
GAMS 1405-FSH |
Y |
Y |
A. Missaoui |
Elite parent; fall dormancy 9 |
3010 |
Y |
Y |
A. Missaoui |
Elite parent; fall dormancy 3 |
CW1010 |
Y |
Y |
A. Missaoui |
Elite parent; fall dormancy 10 |
CUF 101 |
Y |
Y |
D. Samac |
Fall dormancy 10 check |
BIP1 |
Y |
Y |
M. Peel |
Salt tolerant; 27-62 |
BIP2 |
Y |
Y |
M. Peel |
Salt tolerant; 31-6 |
BIP3 |
Y |
Y |
M. Peel |
SemiP; 1-34 |
BIP4 |
Y |
Y |
M. Peel |
SemiP; 6-2 |
BIP5 |
Y |
Y |
M. Peel |
SemiP; 14-46 |
BIP6 |
Y |
Y |
M. Peel |
Drought (Ut7); 17-43 |
BIP7 |
Y |
Y |
M. Peel |
Drought (Ut8); 17-44 |
BIP8 |
Y |
Y |
M. Peel |
Drought (Ut9); 18-22 |
BIP9 |
Y |
Y |
M. Peel |
Drought (Ut10); 21-3 |
BIP10 |
Y |
Y |
M. Peel |
Drought (Ut11); 22-30 |
BIP11 |
Y |
Y |
M. Peel |
Drought (Ut26); 7-18 |
BIP12 |
Y |
Y |
M. Peel |
Drought (Ut30); 13-14 |
Wilson |
N |
Y |
L.-X. Yu |
Elite parent |
WA467895 |
N |
Y |
L.-X. Yu |
Elite parent |
Cornell NY1 |
N |
Y |
D. Viands |
Elite parent |
Cornell NY2 |
N |
Y |
D. Viands |
Elite parent |
Cornell NY3 |
N |
Y |
D. Viands |
Elite parent |
Cornell NY4 |
N |
Y |
D. Viands |
Elite parent |
PAF 13 5, 11-1 |
N |
Y |
H. Riday |
Medicago falcata |
PAF 13 2, 9-4 |
N |
Y |
H. Riday |
Medicago falcata |
PAF 13 9, 10-5 |
N |
Y |
H. Riday |
Medicago falcata |
PAF 13 7, 21-2 |
N |
Y |
H. Riday |
Medicago falcata |
FAL12 1, 11-5 |
N |
Y |
H. Riday |
Medicago falcata |
FAL12 4, 12-4 |
N |
Y |
H. Riday |
Medicago falcata |
MAV8 |
N |
Y |
D. Samac |
Elite parent |
Aph 2 |
N |
Y |
D. Samac |
Elite parent |
MAV13 |
N |
Y |
D. Samac |
Elite parent |
MAV14 |
N |
Y |
D. Samac |
Elite parent |
MAV15 |
N |
Y |
D. Samac |
Elite parent |
ZG9 |
N |
Y |
D. Samac |
Elite parent |
ZG20 |
N |
Y |
D. Samac |
Elite parent |
ZG21 |
N |
Y |
D. Samac |
Elite parent |
ZG23 |
N |
Y |
D. Samac |
Elite parent |
ZG25 |
N |
Y |
D. Samac |
Elite parent |
Aph 11 |
N |
Y |
D. Samac |
Elite parent |
Aph 47 |
N |
Y |
D. Samac |
Elite parent |
PI 516640 |
N |
Y |
B. Irish |
Medicago arabica |
PI 504540 |
N |
Y |
B. Irish |
Medicago arborea |
PI 495215 |
N |
Y |
B. Irish |
Medicago bonarotiana |
PI 315458 |
N |
Y |
B. Irish |
Medicago cancellata |
PI 498767 |
N |
Y |
B. Irish |
Medicago ciliaris |
W6 32886 |
N |
Y |
B. Irish |
Medicago daghestanica |
PI 538998 |
N |
Y |
B. Irish |
Medicago hybrida |
PI 498849 |
N |
Y |
B. Irish |
Medicago laciniata |
PI 537186 |
N |
Y |
B. Irish |
Medicago littoralis |
PI 516711 |
N |
Y |
B. Irish |
Medicago marina |
PI 287999 |
N |
Y |
B. Irish |
Medicago monspelliaca |
PI 537259 |
N |
Y |
B. Irish |
Medicago murex |
PI 220021 |
N |
Y |
B. Irish |
Medicago orbicularis |
PI 464704 |
N |
Y |
B. Irish |
Medicago papillosa |
PI 253450 |
N |
Y |
B. Irish |
Medicago pironae |
W6 5252 |
N |
Y |
B. Irish |
Medicago polymorpha |
PI 150564 |
N |
Y |
B. Irish |
Medicago popovii |
PI 577446 |
N |
Y |
B. Irish |
Medicago prostrata |
PI 631912 |
N |
Y |
B. Irish |
Medicago ruthenica |
PI 631715 |
N |
Y |
B. Irish |
Medicago sativa nothosubsp. tunetana |
PI 631714 |
N |
Y |
B. Irish |
Medicago sativa nothosubsp. tunetana |
PI 631952 |
N |
Y |
B. Irish |
Medicago sativa nothosubsp. varia |
PI 631920 |
N |
Y |
B. Irish |
Medicago sativa nothosubsp. varia |
PI 631923 |
N |
Y |
B. Irish |
Medicago sativa subsp. caerulea |
PI 631921 |
N |
Y |
B. Irish |
Medicago sativa subsp. caerulea |
PI 641405 |
N |
Y |
B. Irish |
Medicago sativa subsp. glomerata |
PI 631978 |
N |
Y |
B. Irish |
Medicago sativa subsp. glomerata |
PI 631869 |
N |
Y |
B. Irish |
Medicago sativa var. viscosa |
PI 631870 |
N |
Y |
B. Irish |
Medicago sativa var. viscosa |
PI 197356 |
N |
Y |
B. Irish |
Medicago scutellata |
I195 x J432 |
N |
Y |
D. Samac |
F1 population (184 progeny) |
AphBC1 |
N |
Y |
D. Samac |
BC1 population (94 progeny) |
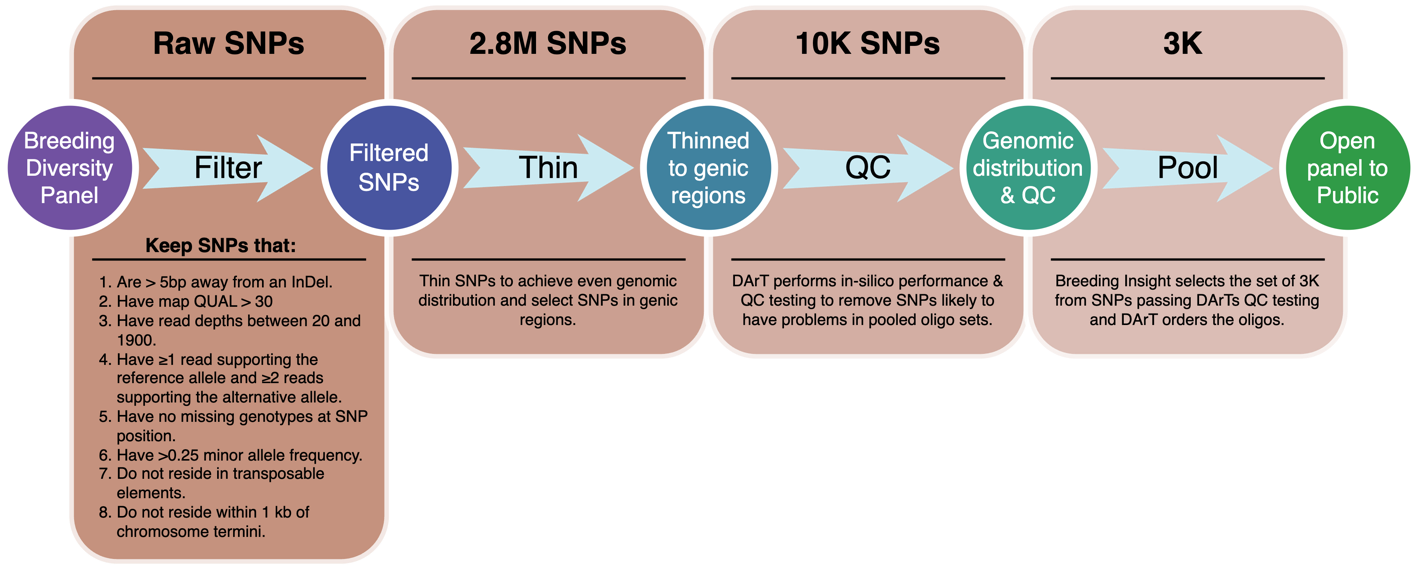
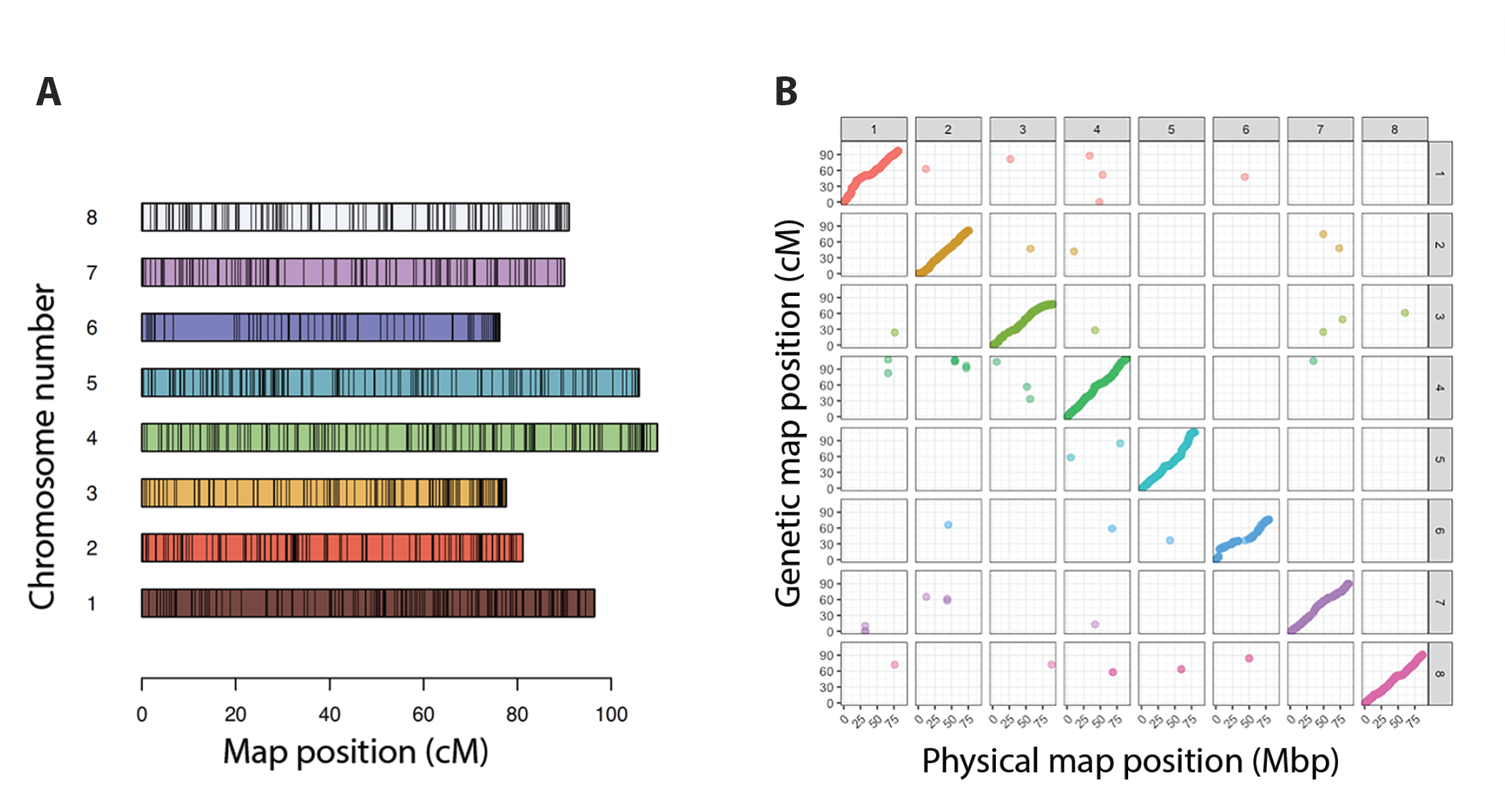
Using the 3K panel genotyping results, we generated linkage maps for two distinct populations, an F1 and a backcross (BC1) that share the parent I195. For the F1 population, individuals were derived from a cross between parents I195 and J432, which are resistant and susceptible to Aphamomyces euteiches, respectively. Meanwhile, the BC1 population was obtained through a cross between I195 and a progeny (85-209) from the above F1 population. Initially, we constructed individual genetic maps for the F1 and BC1 populations. Genotype dosages for both were determined using updog software (Gerard, Ferrão, Garcia, & Stephens, 2018). Subsequently, updog-generated objects were fed into MAPpoly software (Mollinari & Garcia, 2019; Mollinari et al., 2020) to build separate genetic maps for each population. A standard screening was performed based on missing data and Mendelian segregation fit. We calculated the recombination fraction matrix between all retained markers, using this information to cluster markers into linkage groups. According to available genome information, most of these markers corresponded with specific chromosomes. Notably, a few markers that were mapped outside their physical position still presented consistent linkage with the markers in their assigned group. This pattern held true across both F1 and BC1 populations. For each linkage group formed, we used MAPpoly’s functions, mds_mappoly and est_rf_hmm_sequential to carry out de novo ordering and phasing to obtain the final F1 and BC1 maps. From all mapped markers, only 2.55% were assigned to different chromosomes in the F1 map and 0.97% in the BC1 map. After constructing individual maps for the F1 and BC1 populations, we merged them using the genome order (Figure 3A; Supplemental Figure 1A). The mapped markers were all consistent placed in the two maps, but a few markers were assigned to different linkage groups when comparing the linkage and physical assembly in both maps. These markers were retained in filtering because they had reasonable Mendelian segregation behaviour and their association with linkage groups that do not correspond to their physical chromosome assignment could indicate potential errors in the reference assembly (Figure 3B). Markers mapped out of their physical positions were inserted into the genome-based map using the multidimensional scaling (MDS) de novo information. We then reconstructed a joint map by employing the hidden Markov model (HMM) algorithm’s extension, as Mollinari and Garcia (2019) detailed. The implementation for this algorithm can be found in the GitHub repository https://github.com/mmollina/highprecHMM. Finally, haplotypes for all individuals across both F1 and BC1 populations were reconstructed using the same algorithm (Supplemental Figure 1B).
Conclusion
This panel is now publicly available and open for any researcher or breeder to order through DArT (https://www.diversityarrays.com). Researchers interested in using the panel and genotyping services are encouraged to contact DArT directly for pricing details.
Raw data in FASTQ can be requested as can the Missing Allele Discovery File (MADC) that indicates the read depth of each detected haplotype in each sample. The panel and its resulting data are suitable for marker-assisted selection, reconstruction of recombination patterns, allele dosage estimation, and parental confirmation in North American cultivated alfalfa, with some limited application in other Medicago species. The efficacy of the panel on breeding materials outside of North America has not been tested, nor has its efficacy in GWAS. Single plant samples were used to create and test the panel. Subsequent testing on samples that are genotyped individually and in tissue or DNA bulks (DNA bulks up to 30 individuals per population) have produced the same allele frequency ratios in both sample types but higher read depth in pools (Esteban Rios, personal communication). More testing is needed to determine the most efficient number of samples to pool to achieve population-level allele frequencies with minimal human labour and monetary costs.
The DArTag assay can be processed from gDNA or from tissue to genotyping data extraction in a three-week turnaround time. The genotyping data report comprises allele dose calls and raw data with custom report formats available upon request. One benefit that DArTag has over fixed array platforms is the ability to update and improve the panel as required over time. The panel is a pool of 3,000 oligos, one per locus, which is used to generate the sequencing libraries from the assayed material. Because the pool is created from individual oligo stocks, the removal of suboptimal loci or the addition of new loci can be easily done by creating a new pool. To determine which loci should be considered for removal, extensive genotyping (> 10,000 samples) is underway to identify those loci that consistently underperform or fail and flag them for removal. Independently, as new significant QTL markers and/or markers specific to other germplasm are detected, they can be targeted for inclusion in the original pool in the next version(s) of the panel. DArT offers re-pooling services once per year at low or no cost, but more frequent requests could result in labour surcharges being applied (Andrzej Kilian, personal communication). Researchers interested in initiating projects with DArT are encouraged to contact DArT directly for consultation.
Another benefit of the deep testing underway is the ability to detect and catalogue all the microhaplotypes into a fixed allele database, which will improve combining data sets across genotyping projects (manuscript in preparation). If after deep testing it is clear that there are too few markers for GWAS for given traits of interest, additional panels can be made to complement this panel. The other option is to add the required loci to the existing panel up to the technical limit of 7K, which is a more cost-effective option for the routine genotyping service with scalability.
We choose to create a panel of 3,000 loci due to cost and technical reasons, but smaller complementary panels can be made at lower up-front and downstream usage costs. The addition of a complementary 3K panel would nearly double the cost of genotyping per sample but would result in more granular genotyping data.
Data availability statement
The FASTQ files from the whole-genome skim sequencing for the 40 Medicago sativa accessions used for identifying the candidate SNP variants are housed in the NCBI Short Read Archive under the BioProject ID PRJNA1014379. The targeted regions used to create the 3K DArTag markers and the haplotypes detected as of 31 May 2023 (v17) are available on DRYAD (https://datadryad.org/stash/share/wJEn32Dfl94EOYMoeM00PJti6MKUliPBTAtsgbWJyOU). The code and data for construction of the F1, BC1 and joint maps in MAPpoly are available in our GitHub repository for those interested in reproducing our analysis (https://github.com/Breeding-Insight/alfalfa_dartag_panel_paper.git.
Acknowledgments
Breeding Insight is acknowledged for project design, marker development, curation and data processing. Diversity Arrays Technology created the oligo array, provided sequencing services and contributed to the manuscript. We thank S&W Seed Company, Legacy Seeds, Debby Samac, Brian Irish, Michael Peel, Long-Xi Yu, Heathcliffe Riday, Nevin Young, Esteban Rios, Ian Ray, Ali Missaoui and Don Viands for providing germplasm and Sharon Mitchell for manuscript review. Breeding Insight was funded for this work through a Cooperative Agreement between USDA-ARS and Cornell (project number: 8062-21000-043-004-A). Marcelo Mollinari was funded by a USDA NIFA-awarded AFRI grant (project number: 2022-67013-36269).
Supplemental Data
Supplemental Figure 1 . Alfalfa Genetic map construction for an F1, BC1, and a joint map of the consensus.
Supplemental Figure 2. Missing data rates for different grouped subsets of genetic material.
Supplemental Table 1. Final 3,000 loci selected for the DArTag panel.
Author contributions
DZ, KMMG, DS and MJS contributed to experimental design and planning. DZ, DS and MJS selected the diversity panel for WGS. DS, MP and BI grew and harvested all plant materials used in the study. KMMG performed all the WGS analyses, SNP database creation, filtering pipelines and quality control analyses to create the 3K panel. KHU managed the panel creation at Diversity Arrays Technology. DZ, MM and DS executed the data analyses and genetic mapping. DZ and MJS wrote the initial draft of the manuscript. CB managed experiments and communication among all authors involved. All authors contributed to reviewing the manuscript.
Conflict of interest statement
The authors have no conflicts of interest to report.